ChatGPT is probably the most important app released in the past decade. While it started as a chatbot/tech demo for OpenAI's large language models (LLMs), it's now so much more. Its underlying AI models can search the web, generate and evaluate images, and, yes, write silly poems about your boss. And it can also quickly summarize huge documents, generate computer code, and ace tests that few humans can pass. It's now the ultimate multitool.
As OpenAI has added all these features, launched new models, and generally just kept pushing ChatGPT forward, it's become harder and harder to answer what sounds like a simple question: How does ChatGPT work?
Well, I'm going to do my best to answer.
Table of contents:
With Zapier, you can connect ChatGPT to thousands of other apps to bring AI into all your business-critical workflows.
What is ChatGPT?
It's best to think of ChatGPT as an easy-to-use wrapper around some of the most advanced AI models currently available. Its chatbot interface means it can answer your questions, write copy, generate images, draft emails, hold a conversation, brainstorm ideas, explain code in different programming languages, translate natural language to code, solve complex problems, and more—all based on the natural language prompts you feed it. You don't have to jump around between different apps to access its different features—just start up a new conversation in ChatGPT.
Of course, ChatGPT itself relies on AI to determine what model or combination of models is best able to handle your request. Unless you specify a specific AI model, it will automatically pass your prompt on to the one it thinks is most appropriate. As I write this, ChatGPT mostly relies on:
GPT-4o mini and GPT-4o to generate text, process and understand images, and reply quickly.
o1-mini and o1-preview to respond to prompts or questions that require advanced reasoning capabilities.
DALL·E 3 to generate images.
In addition to these primary models, ChatGPT also has:
A voice model that uses GPT-4o to respond to real-time audio queries.
A search model that can search the web, then summarize and cite the most important information.
It's hard to overstate how flexible this combination of AI models is. From the same interface, ChatGPT can write an email to your boss, translate a conversation in real time while you travel, or help you identify a restaurant dish from a photo.
So now that you understand what ChatGPT does (and how much complexity it hides away), let's dig a little deeper into these underlying AI models.
How does ChatGPT work?
The GPT bit of ChatGPT stands for Generative Pre-trained Transformer. Until the release of the OpenAI o1 family of models, all of OpenAI's LLMs and large multimodal models (LMMs) had the GPT-X naming scheme like GPT-4o. But while the names are changing up again, a lot of what's going on under the hood is still largely the same.
The list of concepts I'm about to throw at you might seem a little bit erratic, but they're all important to break down to better understand how LLMs, LMMs, and the other AI models used by ChatGPT work.
Supervised vs. unsupervised learning
A big part of developing AI models is called "training," so let's actually talk about it. The P in GPT stands for "pre-trained," and it's a super important part of why the GPT models are able to do what they can do. The o1 family of models are also pre-trained, as are essentially all other modern AI models, so it's understandable that OpenAI has dropped the P from the naming scheme. It's just not a distinguishing feature any more.
Before GPT-1, the best performing AI models used "supervised learning" to develop their underlying algorithms. They were trained with manually-labeled data, like a database with photos of different animals paired with a text description of each animal written by humans. These kinds of training data, while effective in some circumstances, are incredibly expensive to produce. Even now, there just isn't that much data suitably labeled and categorized to be used to train LLMs.
Instead, GPT-1 employed generative pre-training, where it was given a few ground rules and then fed vast amounts of unlabeled data—near enough the entire open internet. It was then left "unsupervised" to crunch through all this data and develop its own understanding of the rules and relationships that govern text.
As OpenAI continued to develop the pre-training process, it was able to create more powerful models using larger amounts of data, including non-text data. GPT-4o, for example, was trained using the same basic ideas, though in addition to text, its training data also included images and audio. This way, it could learn not only what an apple was, but what one looks like too.
Of course, you don't really know what you're going to get when you use unsupervised learning, so every GPT model is also "fine-tuned" to make its behavior more predictable and appropriate. There are a few ways this is done (which I'll get to), but it often uses forms of supervised learning.
Transformer architecture
All this training is intended to create a deep learning neural network—a complex, many-layered, weighted algorithm modeled after the human brain—which allowed ChatGPT to learn patterns and relationships in the text data and tap into the ability to create human-like responses by predicting what text should come next in any given sentence.
This network uses something called transformer architecture (the T in GPT) and was proposed in a research paper back in 2017. It's absolutely essential to the current boom in AI models.
While it sounds—and is—complicated when you explain it, the transformer model fundamentally simplified how AI algorithms were designed. It allows for the computations to be parallelized (or done at the same time), which means significantly reduced training times. Not only did it make AI models better, but it made them quicker and cheaper to produce.
At the core of transformers is a process called "self-attention." Older recurrent neural networks (RNNs) read text from left-to-right. This is fine when related words and concepts are beside each other, but it makes things complicated when they're at opposite ends of the sentence. (It's also a slow way to compute things as it has to be done sequentially.)
Transformers, however, read every word in a sentence at once and compare each word to all the others. This allows them to direct their "attention" to the most relevant words, no matter where they are in the sentence. And it can be done in parallel on modern computing hardware.
Of course, this is all vastly simplifying things. Transformers don't work with words: they work with "tokens," which are chunks of text or an image encoded as a vector (a number with position and direction). The closer two token-vectors are in space, the more related they are. Similarly, attention is encoded as a vector, which allows transformer-based neural networks to remember important information from earlier in a paragraph.
And that's before we even get into the underlying math of how this works. While it's beyond the scope of this article to get into it, Machine Learning Mastery has a few explainers that dive into the technical side of things.
Tokens
How text is understood by AI models is also important, so let's look a little deeper at tokens. While all data, including images and audio, are also broken down into tokens, the concept is simplest to grasp with text. GPT-3, the original model behind ChatGPT, was trained on roughly 500 billion tokens, which allows its language models to more easily assign meaning and predict plausible follow-on text by mapping them in vector-space.
Many words map to single tokens, though longer or more complex words often break down into multiple tokens. On average, tokens are roughly four characters long. OpenAI has stayed quiet about the inner workings of GPT-4o and o1, but we can safely assume it was trained on at least the same dataset plus as much extra data as OpenAI could access since it's even more powerful.
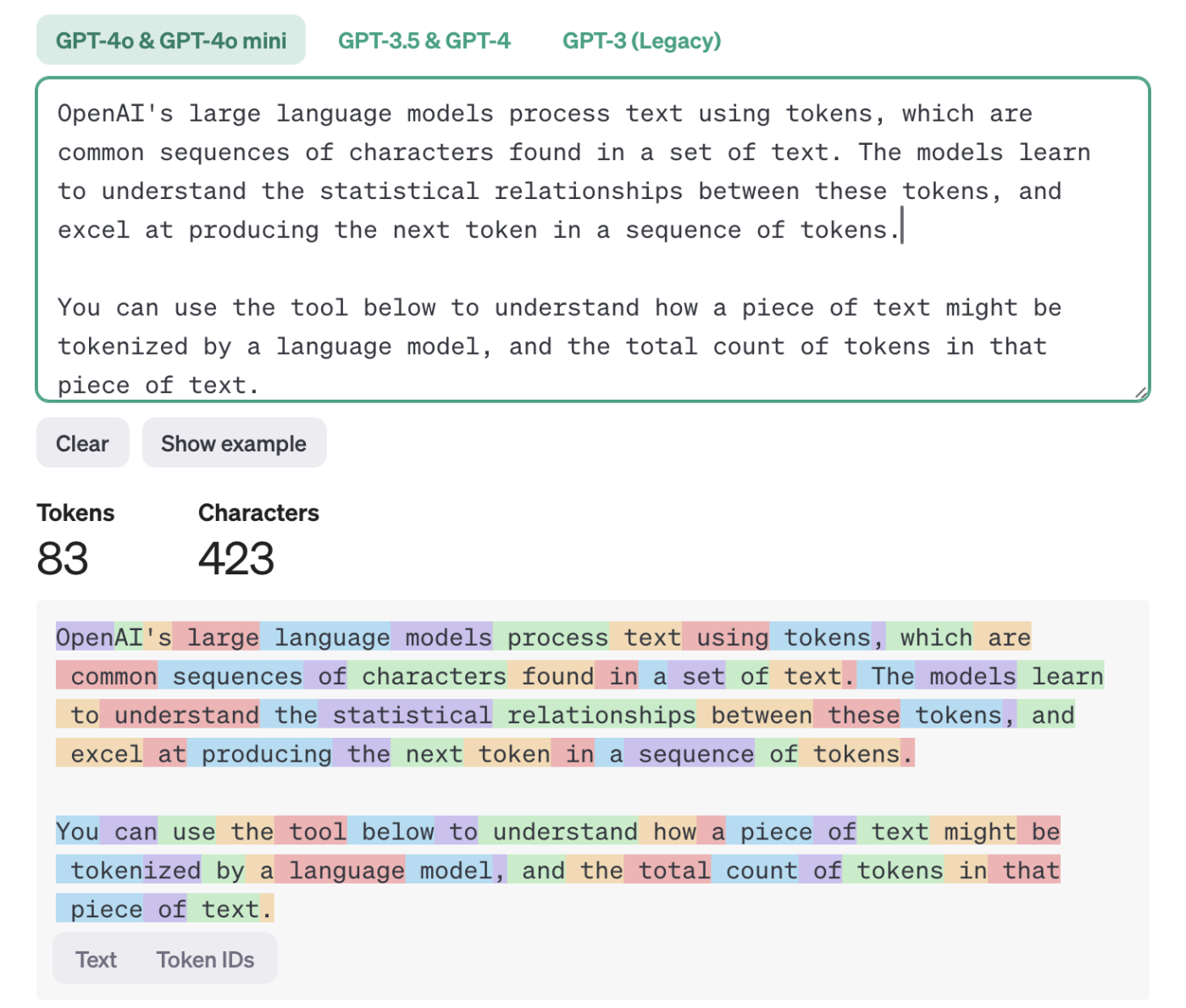
All the text tokens came from a massive corpus of data written by humans, at least for GPT-3. That includes books, articles, and other documents across all different topics, styles, and genres—and an unbelievable amount of content scraped from the open internet. Basically, it was allowed to crunch through the sum total of human knowledge to develop the network it uses to generate text.
Now, researchers are running out of human-created training data, so later models, including o1, are also trained on synthetic—or AI-created—training data. And that's without considering all the image and audio training data that also has to be broken down into discrete tokens.
Based on all that training, GPT-3's neural network has 175 billion parameters or variables that allow it to take an input—your prompt—and then, based on the values and weightings it gives to the different parameters (and a small amount of randomness), output whatever it thinks best matches your request. OpenAI hasn't said how many parameters GPT-4o, GPT-4o mini, or any version of o1 has, but it's a safe guess that it's more than 175 billion and less than the once-rumored 100 trillion parameters, especially when you consider the parameters necessary for additional modalities.
Regardless of the exact number, more parameters doesn't automatically mean better. Some of the developments in model power and performance probably come from having more parameters, but a lot is probably down to improvements in how it was trained.
Unfortunately, the corporate competition between the different AI companies means that their researchers are now unable or unwilling to share all the interesting details about how their models were developed.
Reinforcement learning from human feedback (RLHF)
Without further training, any LLM's neural network is entirely unsuitable for public release. GPT was trained on the open internet with almost no guidance, after all—can you imagine the horrors?
So, to further refine its model's abilities to respond to a variety of different prompts in a safe, sensible, effective, and coherent way, they were optimized with a technique called reinforcement learning with human feedback (RLHF).
Essentially, OpenAI created some demonstration data that showed the neural network how it should respond in typical situations. From that, they created a reward model with comparison data (where two or more model responses were ranked by AI trainers) so the AI could learn which was the best response in any given situation. While not pure supervised learning, RLHF allows networks like GPT to be fine-tuned effectively.
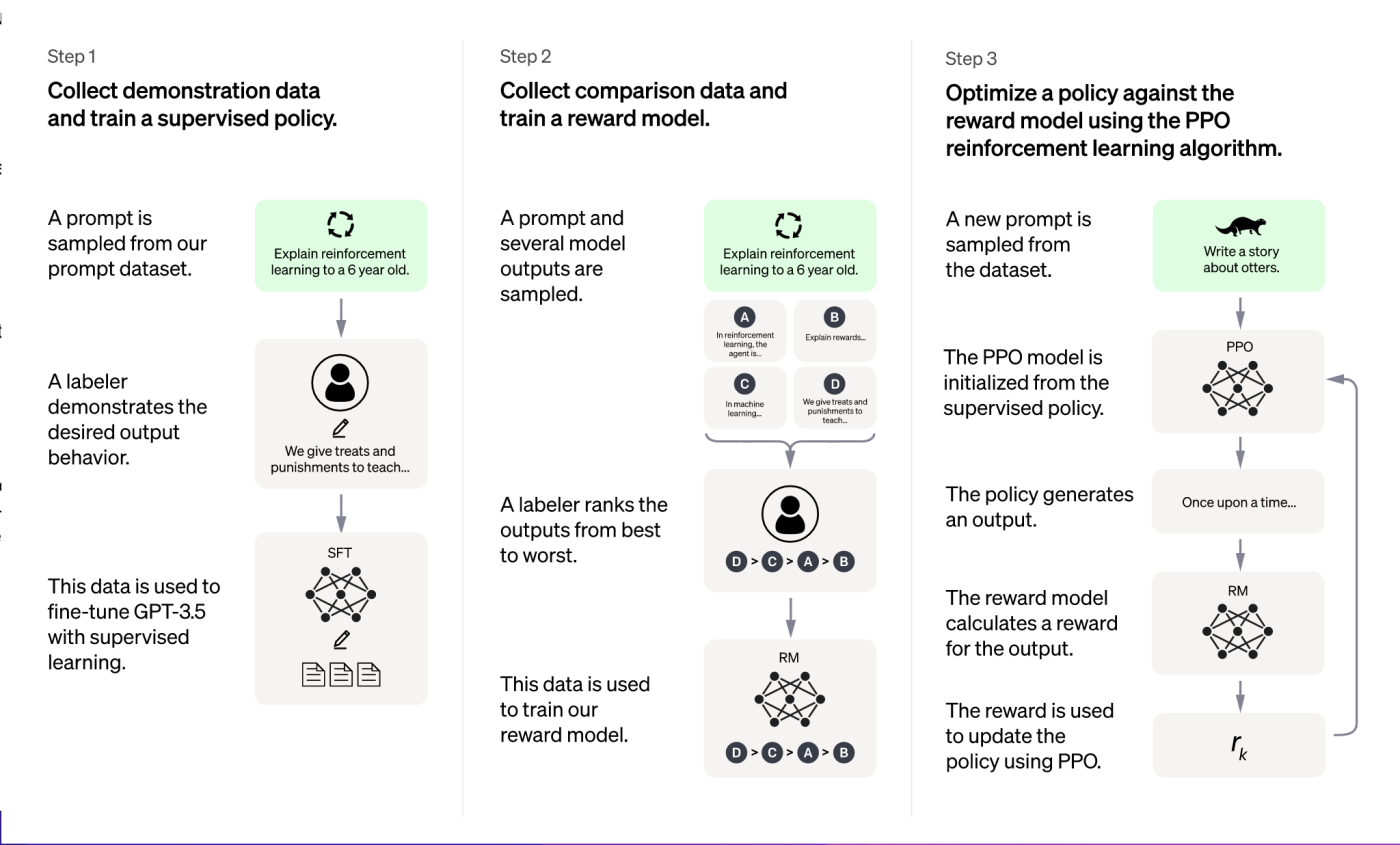
Reinforcement learning is used to make AI models safer—by steering them away from harmful and biased responses—and to make them more effective—by optimizing them for human-like dialog. Advances in reinforcement learning allow each generation of models to be safer and more reliable.
In particular, the o1 family of models was trained using reinforcement learning to reason through problems using a technique called chain-of-thought (CoT).
Chain-of-thought reasoning (CoT)
LLMs like GPT-4o struggle with complex, multi-step problems. Their training cues them to respond to most challenges with the simple and obvious answer, not some wild supposition. When you ask ChatGPT to write you an email, you don't want it to do it in Morse code on a whim.
But by defaulting to the obvious response, LLMs aren't great at advanced logic puzzles, hard math, and other kinds of problems that require multiple steps. This is where CoT reasoning comes in.
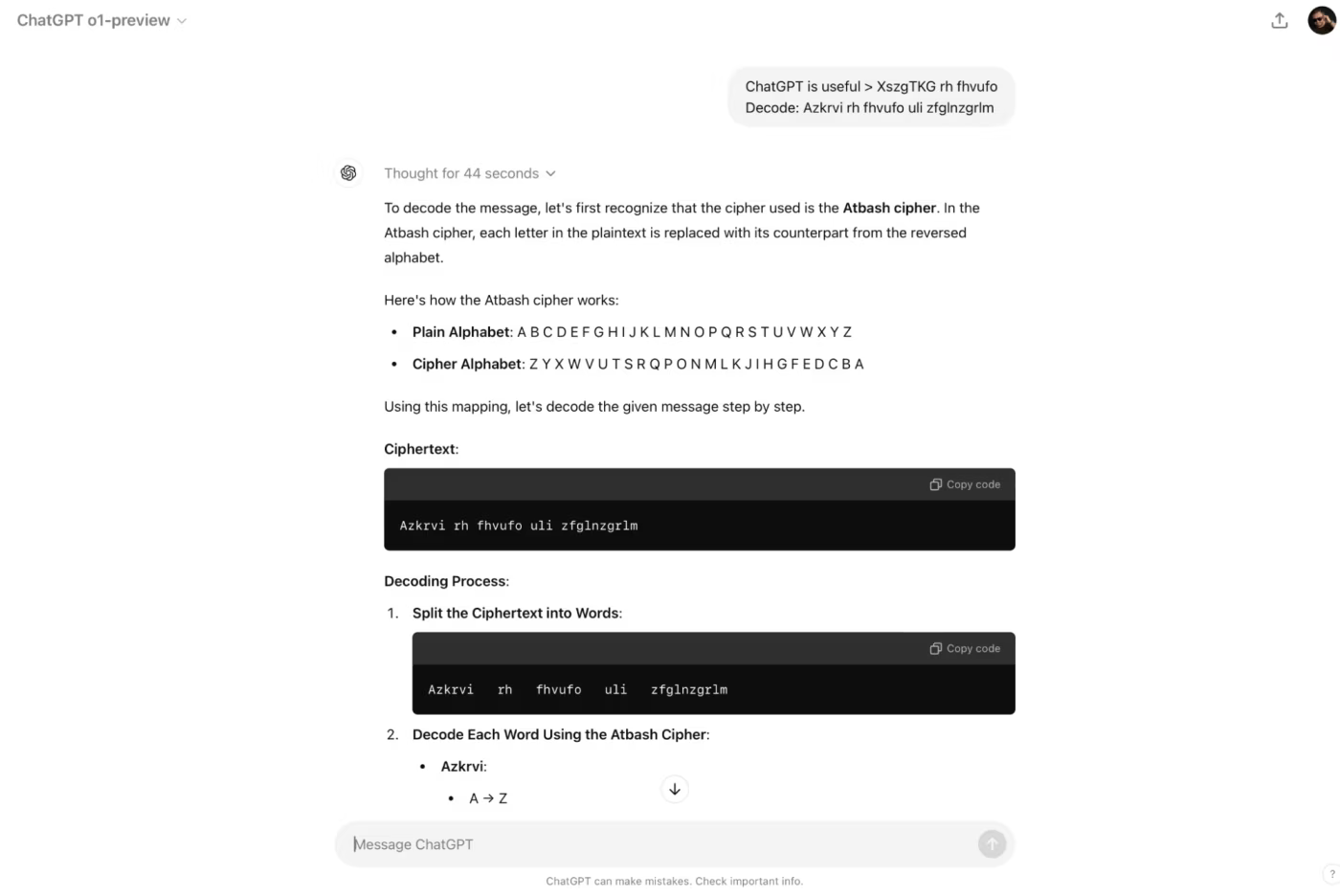
The o1 model was trained in such a way that it's able to break problems down into their constituent parts. If you ask it to solve a cipher or logic puzzle, it will take time to assess the problem, try multiple solutions, and work through it, rather than just responding with the first plausible set of text. Crucially, CoT reasoning takes time and additional computing resources, so ChatGPT only uses o1 for prompts that call for it.
Unfortunately, OpenAI is keeping the details quiet about how they accomplished this, but you can read a bit more in this deep dive into the o1 model family.
Natural language processing (NLP)
All this effort is intended to make OpenAI's models as effective as possible at natural language processing (NLP). NLP is a huge bucket category that encompasses many aspects of artificial intelligence, including speech recognition, machine translation, and chatbots, but it can be understood as the process through which Al is taught to understand the rules and syntax of language, programmed to develop complex algorithms to represent those rules, and then made to use those algorithms to carry out specific tasks.
Since I've covered the training and algorithm development side of things, let's look at how NLP enables GPT to carry out certain tasks—in particular, responding to text-based user prompts. (We'll look at other kinds of prompts in a moment.)
It's important to understand that for all this discussion of tokens, ChatGPT is generating text based on what words, sentences, and even paragraphs or stanzas could follow. It's not the predictive text on your phone bluntly guessing the next word; it's attempting to create fully coherent responses to any prompt. This is what transformers bring to NLP.
In the end, the simplest way to imagine it is like one of those "finish the sentence" games you played as a kid.
In the end, the simplest way to imagine it is like one of those "finish the sentence" games you played as a kid. ChatGPT starts by taking your prompt, breaking it down into tokens, and then using its transformer-based neural network to try to understand what the most salient parts of it are, and what you are really asking it to do. From there, the neural network kicks into gear again and generates an appropriate output sequence of tokens, relying on what it learned from its training data and fine-tuning.
For example, when I gave ChatGPT the prompt, "Zapier is…" it responded saying:
"Zapier is an online automation tool that helps connect different applications and services without the need for coding."
That's the kind of sentence you can find in hundreds of articles describing what Zapier does, so it makes sense that it's the kind of thing that it spits out here. But when my editor gave it the same prompt, it said:
"Zapier is an online automation tool that connects your apps and services, enabling you to automate repetitive tasks without coding or relying on developers."
That's pretty similar, but it isn't exactly the same response. Asking "What is Zapier?", "What does Zapier do?", and "Describe Zapier" all get similar results too, presumably because they occupy similar positions in vector space. GPT understands that the most salient word here is Zapier, and that all the others are just asking for a short summary in slightly different ways.
That randomness (which you can control in some GPT apps with a setting called "temperature") ensures that ChatGPT isn't just responding to every single response with what amounts to a stock answer. It's running each prompt through the entire neural network each time, and rolling a couple of dice here and there to keep things fresh. Its understanding of natural language also allows it to parse the subtle differences between "What is Zapier?" and "What does Zapier do?" While fundamentally similar questions, you would expect the answer to be slightly different. Whatever way you ask things, ChatGPT is not likely to start claiming that Zapier is a color from Mars, but it will mix up the following words based on their relative likelihoods.
Multimodality in ChatGPT
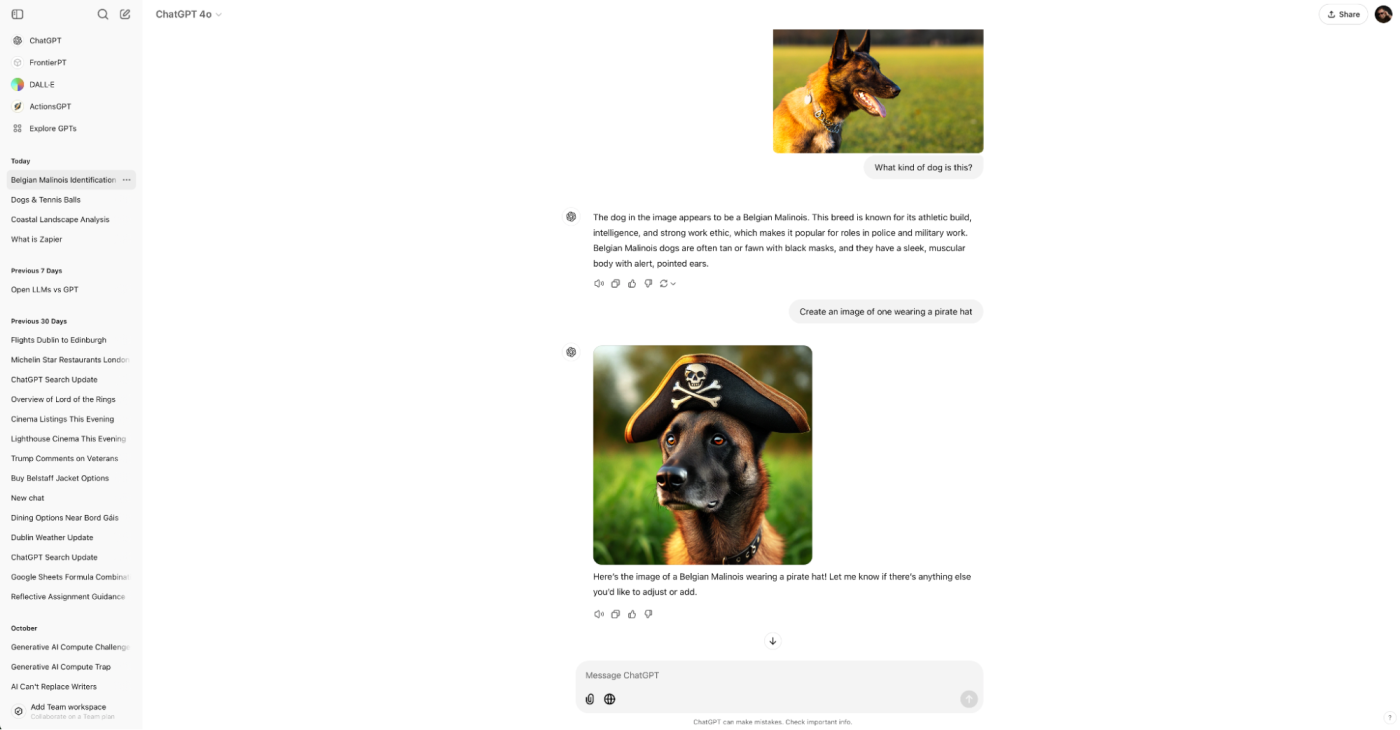
While natural language processing is the defining feature of ChatGPT, it's also multimodal. That means that ChatGPT can understand text, images, and audio (among other inputs) as part of the same prompt.
This is what allows it to process documents and images, parse graphs and charts, or respond to your request with an image from DALL·E 3. It's also what enables the advanced voice mode on the ChatGPT mobile app that you can just chat away to, and even interrupt.
While the development process for all these additional features is incredibly complex, they still rely on a lot of the same AI building blocks of transformers, tokens, and training.
Extensibility in ChatGPT
One of the most powerful aspects of ChatGPT is that it's no longer just a chatbot with a limited set of knowledge based on its training data. Here are some of the most powerful ways you can use ChatGPT:
The desktop app allows you to access ChatGPT at any time. It can see content on your screen and can now work with coding apps.
The mobile app allows you to use advanced voice mode with ChatGPT as well as upload photos directly from your phone.
ChatGPT Search allows you to find real-time information from the web in ChatGPT.
GPTs let you build your own customized bots on ChatGPT.
Zapier's ChatGPT integration lets you connect ChatGPT to thousands of other apps, so you can get ChatGPT content from whatever app you're in.
What is the ChatGPT API?
OpenAI doesn't have a just-us attitude with its technology. The company has an API platform that allows developers to integrate the power of ChatGPT into their own apps and services (for a price, of course).
Zapier uses the ChatGPT API to power its own ChatGPT integration, which lets you connect ChatGPT to thousands of other apps and add AI to your business-critical workflows. Learn more about how to automate ChatGPT, or take a look at these examples to get you started.
Create email copy with ChatGPT from new Gmail emails and save as drafts in Gmail
Start a conversation with ChatGPT when a prompt is posted in a particular Slack channel
Generate conversations in ChatGPT with new emails in Gmail
Zapier is the leader in workflow automation—integrating with thousands of apps from partners like Google, Salesforce, and Microsoft. Use interfaces, data tables, and logic to build secure, automated systems for your business-critical workflows across your organization's technology stack. Learn more.
What's next for ChatGPT?
ChatGPT has gone from a tech demo and a novelty to an increasingly useful productivity tool in two short years. Its multimodality, advanced reasoning using the o1 models, and integrations with other apps make it among the most powerful AI tools available.
As for what's next? OpenAI is almost certainly going to continue to update existing models and build new ones. As that happens, expect ChatGPT's capabilities to leap forward again and again.
Related reading:
This article was originally published in February 2023. The most recent update was in November 2024.