Business tips
7 min readStructured vs. unstructured data: What's the difference?
By Michael Kern · September 7, 2023
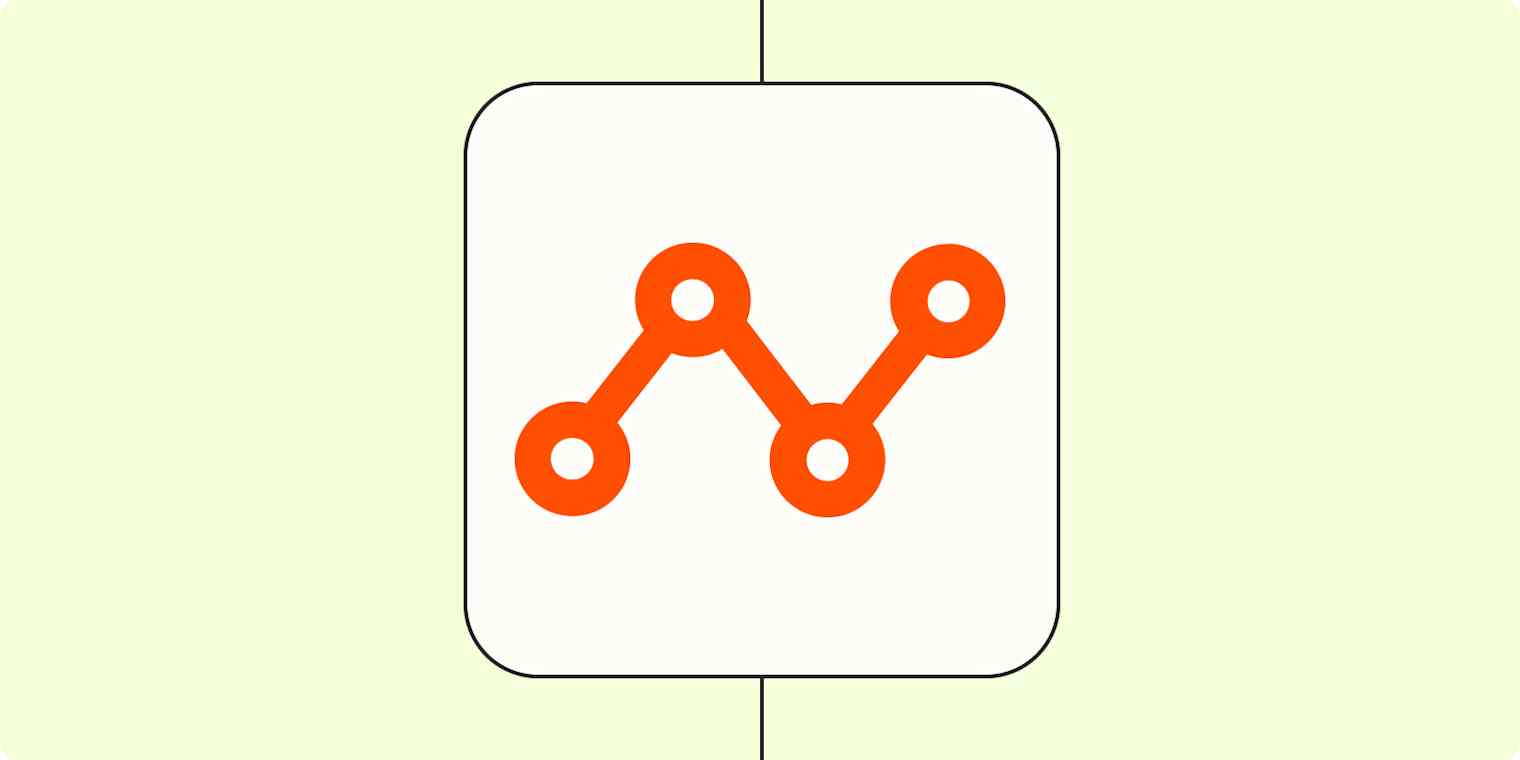
Get productivity tips delivered straight to your inbox
We’ll email you 1-3 times per week—and never share your information.
Related articles
Improve your productivity automatically. Use Zapier to get your apps working together.
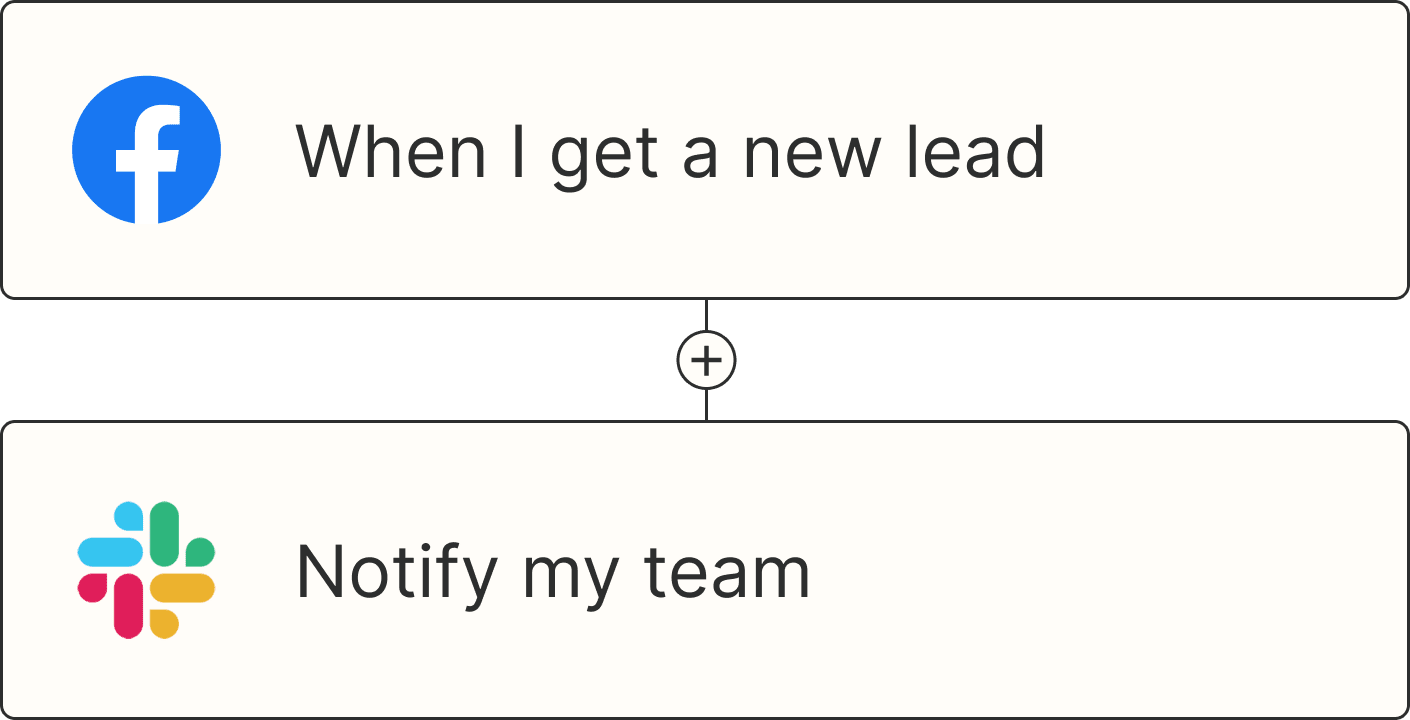